"We are all forecasters. When we think about changing jobs, getting married, buying a home, making an investment, launching a product, or retiring, we decide based on how we expect the future will unfold. These expectations are forecasts."
Section: 1, Chapter: 1
Philip Tetlock considers himself an "optimistic skeptic" when it comes to forecasting. The skeptical side recognizes the huge challenges of predicting the future in a complex, nonlinear world. Even small unpredictable events, like the self-immolation of a Tunisian fruit vendor, can have cascading consequences no one foresaw, like the Arab Spring uprisings.
However, the optimistic side believes foresight is possible, to some degree, in some circumstances. We make mundane forecasts constantly in everyday life. Sophisticated forecasts underpin things like insurance and inventory management. The key is to figure out what makes forecasts more or less accurate, by gathering many forecasts, measuring accuracy, and rigorously analyzing results. This is rarely done today - but it can be.
Section: 1, Chapter: 1
The luck-skill continuum is a powerful conceptual model for understanding the relative contribution of luck and skill in different domains. The key features of the continuum are:
- The extreme left represents pure luck (e.g. roulette) and the extreme right represents pure skill (e.g. chess)
- Most activities fall somewhere between these extremes, combining both luck and skill
- As you move from right to left, luck plays an increasingly important role and larger sample sizes are needed to detect skill
- Reversion to the mean is stronger on the left (luck) side of the continuum
- Differences in skill are easier to observe on the right side of the continuum
The continuum is useful for setting expectations and making better decisions. If you know where an activity lies on the continuum, you can better interpret past results and anticipate future outcomes.
Section: 1, Chapter: 3
Mauboussin offers practical advice on how to use the concept of reversion to the mean to make better predictions and decisions:
- Reversion to the mean is a statistical reality in any system where two measures are imperfectly correlated. Extreme outcomes tend to be followed by more average outcomes.
- The key to using reversion to the mean is to know where the mean is. In other words, you need a sense of the underlying base rate or long-term average. Err on the side of the mean.
- The more extreme the initial outcome and the further it is from the mean, the more you should expect it to revert.
Section: 1, Chapter: 10
The ideal decision group for debiasing and improving choices has the following traits:
- A commitment to rewarding and encouraging truthseeking, objectivity and openness
- Accountability - members must know they'll have to explain their choices to the group
- Diversity of perspectives to combat groupthink and confirmation bias
The group can't just be an echo chamber. There must be a culture of rewarding dissent, considering alternatives, and constantly asking how members might be wrong or biased. If you can find even 2-3 other people who share this ethos, you'll be far ahead of most decision makers.
Section: 1, Chapter: 4
The flipside of a premortem is "backcasting" - envisioning a successful outcome, then reverse-engineering how you got there. If your company wants to double its market share, imagine it's five years from now and that's been accomplished. What key decisions and milestones led to that rosy future?
Telling the story of success makes it feel more tangible. It also helps identify must-have elements that might otherwise be overlooked. Backcasting is a great technique for setting and pressure-testing goals. Use it for anything from launching products to planning vacations.
Section: 1, Chapter: 6
A classic example of the danger of judging decisions solely by their results is the rise in obesity that accompanied the low-fat diet craze. Public health officials encouraged people to shun fatty foods and embrace carbs and sugars instead in the 1980s-90s. But obesity skyrocketed as a result.
However, in the moment, people eating "low-fat" but high-sugar snacks like SnackWells cookies likely attributed any weight gain to bad luck or other factors. It took a long time for the realization that judging food quality by fat content alone was flawed. This shows the peril of "resulting" - assuming the quality of a decision can be judged solely by its outcome.
Section: 1, Chapter: 3
Chapter 5 explores how great decision-making groups don't just tolerate dissent, they actively encourage it. Duke cites the example of Alfred P. Sloan, the legendary CEO of General Motors. When all his executives agreed on a decision, Sloan said "I propose we postpone further discussion of this matter until our next meeting to give ourselves time to develop disagreement and perhaps gain some understanding of what the decision is all about." He knew that the pursuit of truth required constantly stress-testing ideas and considering alternatives. Dissent isn't disloyal, it's necessary for getting to the best answer.
Section: 1, Chapter: 5
In Chapter 1, Annie Duke argues that life is more like poker than chess. Chess contains no hidden information and little luck, so the better player almost always wins. But in poker and in life, there are unknown variables and luck involved. Even the best decision doesn't always lead to a good outcome, and a bad decision can sometimes work out due to luck. Duke uses examples like Pete Carroll's famous goal-line call in the Super Bowl and how the same play call would have been deemed brilliant rather than idiotic if it had worked.
Section: 1, Chapter: 1
"Outcomes don't tell us what's our fault and what isn't, what we should take credit for and what we shouldn't. Outcomes are rarely the result of our decision quality alone or chance alone, and outcome quality is not a perfect indicator of the influence of luck or skill. When it comes to fielding outcomes, we tend to focus on the quality of the outcome as the deciding factor between luck and skill."
Section: 1, Chapter: 3
One habit that aids truthseeking discussions, both in groups and one-on-one, is expressing uncertainty. Rather than stating opinions as facts, couch them in probabilistic terms. Say things like "I think there's a 60% chance that..." or "I'm pretty sure that X is the case, but I'm open to other views." Expressing uncertainty:
- Acknowledges that reality is complex and our knowledge is limited
- Makes people more willing to share dissenting opinions
- Sets the stage for you to change your mind gracefully if better evidence emerges
Expressing certainty, on the other hand, cuts off discussion and makes you look foolish if you're wrong. It's a lazy way to "win" arguments.
Section: 1, Chapter: 6
Chapter 3 focuses on how to learn productively from outcomes. Duke argues we must get better at "fielding" outcomes - determining whether they were due to the quality of our decisions (skill) or factors beyond our control (luck).
Poker players know that even good decisions can lead to bad outcomes and vice versa due to luck. The challenge is that it's hard to tease apart the contributions of luck vs. skill. But if we attribute bad outcomes solely to luck, we miss opportunities to improve our choices. If we chalk up good outcomes solely to skill, we may wrongly reinforce bad habits.
Section: 1, Chapter: 3
One of the key insights is that we should get more comfortable saying "I'm not sure" and acknowledging uncertainty. Poker players know that they can never be fully certain if their decision is right, due to incomplete information. They focus on making the best decision possible given what they know. We should do the same in life - make the best choices we can while accepting that we don't know everything. Don't be afraid to express uncertainty, as it makes you more credible. Redefine "wrong" to mean the decision-making process was flawed, not that the outcome was bad due to factors beyond your control.
Section: 1, Chapter: 1
To create a truthseeking culture, Duke recommends following the Mertonian norms developed by sociologist Robert Merton:
- Communism (data belongs to the group, not individuals)
- Universalism (evaluate ideas based on merit, not source)
- Disinterestedness (be willing to accept outcomes that go against your preferred position)
- Organized Skepticism (discussion is good, but agree to be bound by logic/evidence)
These principles help overcome biases like confirmation bias, motivated reasoning, and groupthink that can derail group decision making. They create an environment where the best ideas can surface and win out.
Section: 1, Chapter: 5
One way to harness the power of dissent is to conduct a "premortem" on important decisions. A premortem involves imagining a future where your plan failed, then working backwards to figure out why.
Have the team brainstorm as many paths to failure as possible - imagine competitors' responses, think through operational snafus, consider external risks. Then update your plan to mitigate the identified issues. This "creative dissent" makes the final plan much more robust. Premortems give permission to express doubts in a productive way.
Section: 1, Chapter: 6
Ideally, we would just be able to recognize and overcome biases like self-serving bias through sheer force of will. But these patterns of thinking are so ingrained that individual willpower is rarely enough to change them. A better solution is to recruit others to help us see our blind spots.
Surround yourself with people who are on a "truthseeking" mission and aren't afraid to challenge you if your fielding of outcomes seems biased. Ideally, gather a group with diverse perspectives who are all committed to being open-minded, giving credit where due, and exploring alternative interpretations of events. Use them to vet your decision-making process, not just focus on outcomes.
Section: 1, Chapter: 4
One of the key themes of Chapter 4 is that making better, less biased decisions is a learnable skill, not an innate ability. You can create habits and routines that will gradually improve your "batting average" on choices, even if it feels uncomfortable and unnatural at first.
Part of developing this skill is learning strategies for anticipating common decision traps, so you can spot them ahead of time and circumvent them. Groups can play a huge role by helping you catch flaws in your process in a timely way. Don't expect perfection, just aim to get a little more rational and objective over time. Those gains will compound.
Section: 1, Chapter: 4
Even highly-educated experts like doctors aren't immune to decision-making biases. When a patient presents with a cough, the doctor has to decide if it's due to a virus, allergies, acid reflux, cancer or other causes. Leaping to conclusions based on initial impressions leads to a lot of misdiagnoses.
That's why many medical facilities use checklists, group consultations and decision aids to debias doctors. The Kaiser health system reduced the number of patients on strong opioids by 60% by having another doctor review any long-term painkiller prescription. The outside perspective combatted the prescribing doctor's faulty pattern-matching.
Section: 1, Chapter: 4
Annie Duke introduces the powerful question "Wanna bet?" as a way to test the strength of your beliefs. When someone challenges you with a bet, it forces you to consider:
- Why do I believe this?
- How much information do I have to support it?
- Under what circumstances might my belief not be true?
"Wanna bet?" triggers you to vet your beliefs and express your level of confidence in them accurately, rather than just assuming they are 100% true. This is what poker players and good decision makers do constantly. Duke argues we should all adopt this betting framework for our beliefs and predictions.
Section: 1, Chapter: 2
Duke cites research showing that we form abstract beliefs in a backwards way - we hear something, assume it's true, and only sometimes get around to vetting it later, if at all.
Experiments found that even when questionable information was clearly labeled as false, people still tended to process it as true, especially when under time pressure. Our brains evolved to assume things we hear are true because doubting everything would be cognitively inefficient. But this means many of our beliefs about the world are not properly vetted. Even when presented with contradictory evidence, we often still cling to existing beliefs.
Section: 1, Chapter: 2
A core theme of Chapters 5-6 is that mentally simulating the future and past leads to better choices. Vividly imagining various futures (through backcasting and premortems) helps us select the most promising one to aim for. It also allows us to anticipate and preempt obstacles. Reflecting on past similar situations provides context on whether a proposed course of action is wise.
The more we build mental muscles to escape the here-and-now and adopt a long-term perspective, the better our judgment will be. Groups and habits that promote this kind of mental time travel are invaluable.
Section: 1, Chapter: 6
Silver advocates for a Bayesian approach to prediction and belief-formation. Bayes's theorem states that we should constantly update our probability estimates based on new information, weighing it against our prior assumptions. Some key takeaways:
- Explicitly quantify how probable you think something is before looking at new evidence. This prevents the common error of assigning far too much weight to a small amount of new data.
- Think probabilistically, not in binary terms. Assign levels of confidence to your beliefs rather than 100% certainty or 0% impossibility.
- Be willing to change your mind incrementally based on new information. Don't cling stubbornly to prior beliefs in the face of mounting contradictory evidence.
- Aim to steadily get closer to the truth rather than achieving perfection or claiming to have absolute knowledge. All knowledge is uncertain and subject to revision.
Section: 1, Chapter: 8
There are two main approaches to solving "optimal stopping" problems like hiring employees or buying a house:
- Look-Then-Leap Rule: Gather information for a set period of time, then commit to the next option that exceeds the best you've seen. This is the 37% Rule.
- Threshold Rule: If you have full prior information, simply set a predetermined threshold for quality and immediately take the first option that meets or exceeds it. No "look" phase needed.
The Threshold Rule only works if you have solid information on the distribution of options before you start looking. For example, if you know the distribution of typing speeds for secretaries, you can set a threshold and hire the first applicant who meets it. If you lack that information, the Look-Then-Leap approach is necessary to first establish a baseline.
In many real-world scenarios, from buying a house to choosing a spouse, we lack reliable priors. So some initial exploration, per the 37% Rule, is optimal before setting a threshold to leap for. The more uncertainty, the more exploration is needed before exploiting.
Section: 1, Chapter: 1
Imagine you need to decide which employee to promote or which vendor to source from. You want to be scrupulously fair, but you're concerned that subconscious biases might creep in. Randomness offers an unexpected solution. Rather than agonizing to perfectly weigh every factor, you can:
- Set a clear quality bar for eligibility. Decide what minimum standards candidates must meet to be considered qualified.
- Make a binary yes/no decision on each candidate. No rankings, no attempt to suss out who's better by how much. Just "above the bar" or not.
- Among all candidates who clear the bar, choose one at random.
This process, while not perfect, removes many common flaws and biases from selection:
- Leniency bias (grading too easily) gets blunted because you're working off clear standards, not gut feeling
- Recency bias (overweighting latest information) is thwarted, since you decide on each candidate one at a time rather than comparing
- Implicit biases get less room to operate since all "above the bar" options are treated as equal
Randomness becomes a guarantor of equity rather than a bug.
Section: 1, Chapter: 9
A key insight from the multi-armed bandit problem is the power of "optimism in the face of uncertainty." That is, when choosing between options where some information is known and some unknown, optimism is the mathematically correct approach.
Suppose you walk into a casino and see two slot machines. The first, you're told, pays out 20% of the time. The second machine's payoff rate is unknown. Which should you choose?
Rationally, you should try the mystery machine. That's because it COULD pay out at >20%, in which case it's the better choice. But you'll only find out if you try it. Mathematically, the expected value of an unknown option is higher than a known suboptimal one.
So in life, when facing uncertainty, choose optimistically - assume the best of a new person, place, or experience. Optimism maximizes your chance of finding something great. Pessimism can lead to overlooking hidden gems.
Section: 1, Chapter: 2
The explore/exploit tradeoff, also known as the multi-armed bandit problem, offers guidance on when to stop exploring new options and commit to the best known one. The optimal approach depends on the total length of time you'll be making decisions:
- Short time horizon (e.g. choosing where to eat on your last night of vacation): Exploit immediately by picking the best place you've been to already. Don't risk a bad meal to explore.
- Medium time horizon (e.g. choosing lunch spots in the few months before moving to a new city): Mix it up between exploiting old favorites and exploring to find new ones. Lean more toward exploring early on.
- Long time horizon (e.g. choosing jobs or relationships when young): Explore a lot, try new things constantly, don't settle down too soon. With decades of decisions ahead, finding a great option is worth many misses.
Section: 1, Chapter: 2
What's the optimal balance between exploring new options and exploiting known ones? Mathematician John Gittins solved this in the 1970s with the Gittins Index.
The Gittins Index assigns each option a score based on its observed results so far AND the uncertainty remaining in that option. Unknown options get an "uncertainty bonus" that makes them more attractive to try.
For example, suppose you have two slot machines, one that paid off 4/10 times, and a new machine you've never tried. The Gittins Index will recommend the new machine, because the uncertainty bonus outweighs the 40% payoff of the known machine. It COULD be much better.
Once you've tried an option enough times, the uncertainty bonus dwindles and its Gittins Index matches its observed performance. At that point you "retire" an option that underperforms.
Section: 1, Chapter: 2
When deciding what to keep and what to discard, whether it's for your closet, your bookshelf, or your computer's memory, consider two factors:
- Frequency: How often is this item used or accessed? Things used most often should be kept close at hand. This is why your computer's RAM is faster than its hard disk.
- Recency: When was this item last used? Items used more recently are more likely to be used again soon. So the most recently used items should also be kept easily accessible.
Many caching algorithms, like Least Recently Used (LRU), primarily consider recency. But the optimal approach, as used by the human brain, balances both frequency and recency.
Section: 1, Chapter: 4
The 37% Rule provides guidance on the optimal time to stop searching and commit to a particular choice, whether you're looking for an apartment, hiring an employee, or finding a spouse. In short, look at your first 37% of options to establish a baseline, then commit to anything after that point which beats the best you've seen so far.
To be precise, the optimal proportion to look at before switching to "leap mode" is 1/e, or about 37%. So if you're searching for an apartment and have 30 days to do it, spend the first 11 days (37% of 30) exploring options, then on day 12 pick the next place that tops your current best.
This algorithm offers the best chance of finding the single best option, though it will still fail a majority of the time. But it shows the power of establishing a "good enough" baseline before jumping on something that exceeds it.
Section: 1, Chapter: 1
Books about Decision Making
Prediction
Decision Making
Thinking
Leadership
Superforecasting Book Summary
Philip Tetlock
In Superforecasting, Philip Tetlock and Dan Gardner reveal the techniques used by elite forecasters to predict future events with remarkable accuracy, and show how these skills can be cultivated by anyone to make better decisions in an uncertain world.
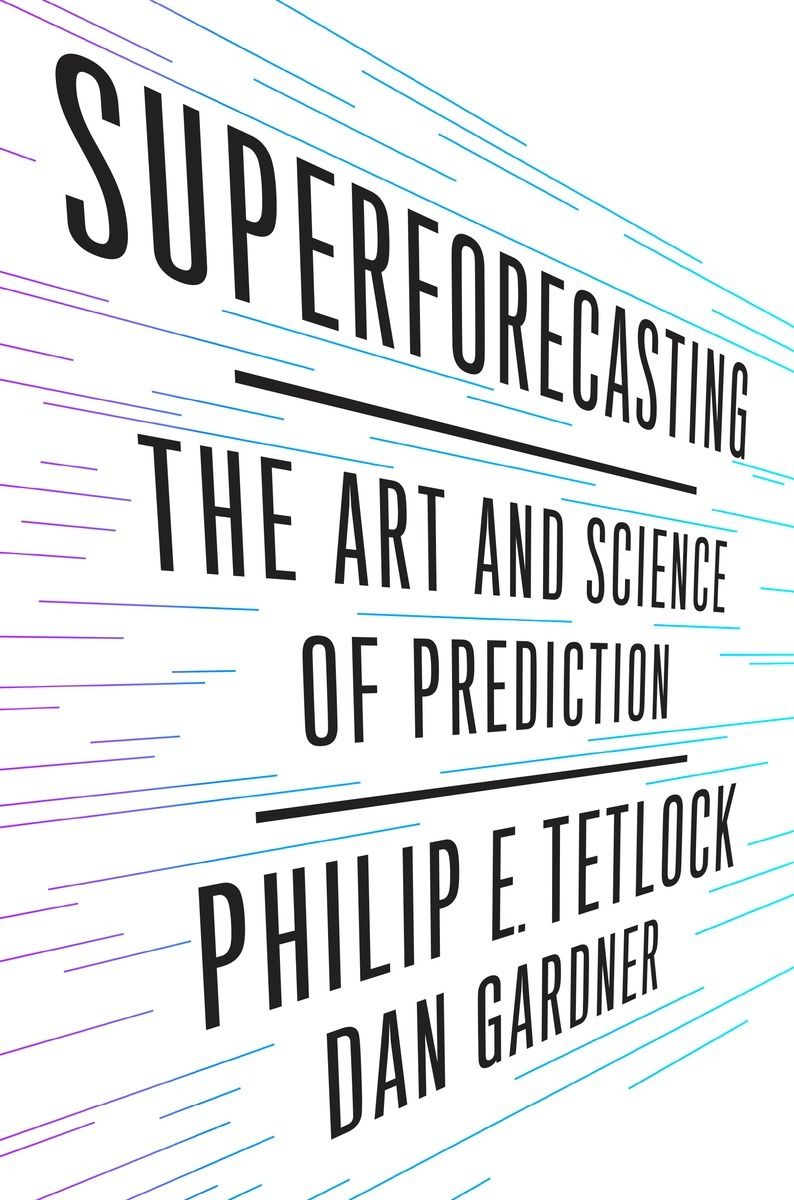
Sports
Management
Psychology
Decision Making
The Success Equation Book Summary
Michael Mauboussin
The Success Equation is a comprehensive guide to understanding the relative roles of skill and luck in shaping outcomes, offering practical insights and tools for improving decision-making, performance, and predictions in a wide range of domains, from sports and business to education and investing.
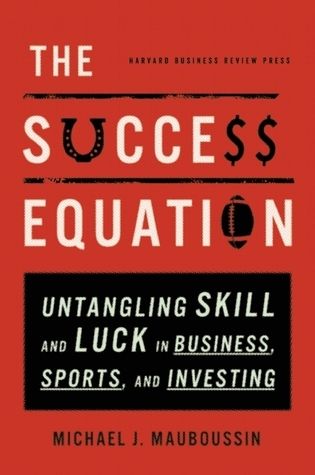
Personal Development
Decision Making
Psychology
Thinking in Bets Book Summary
Annie Duke
In "Thinking in Bets," Annie Duke draws on her experience as a professional poker player to share strategies for making sound decisions under uncertainty, such as thinking probabilistically, learning from outcomes, surrounding yourself with truthseeking groups, and using mental time travel to pressure-test beliefs and plans.
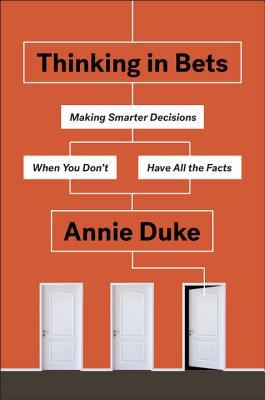
Prediction
Decision Making
Economics
The Signal and the Noise Book Summary
Nate Silver
In The Signal and the Noise, Nate Silver explores the art and science of prediction, explaining what separates good forecasters from bad ones and how we can all improve our understanding of an uncertain world.
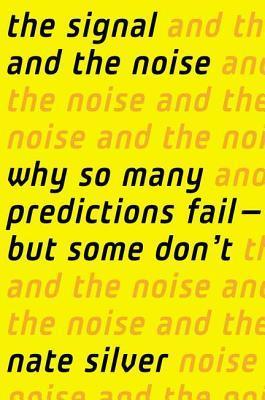
Computer Science
Decision Making
Algorithms To Live By Book Summary
Brian Christian
Algorithms to Live By reveals how computer algorithms can solve many of life's most vexing human problems, from finding a spouse to folding laundry, by providing a blueprint for optimizing everyday decisions through the lens of computer science.
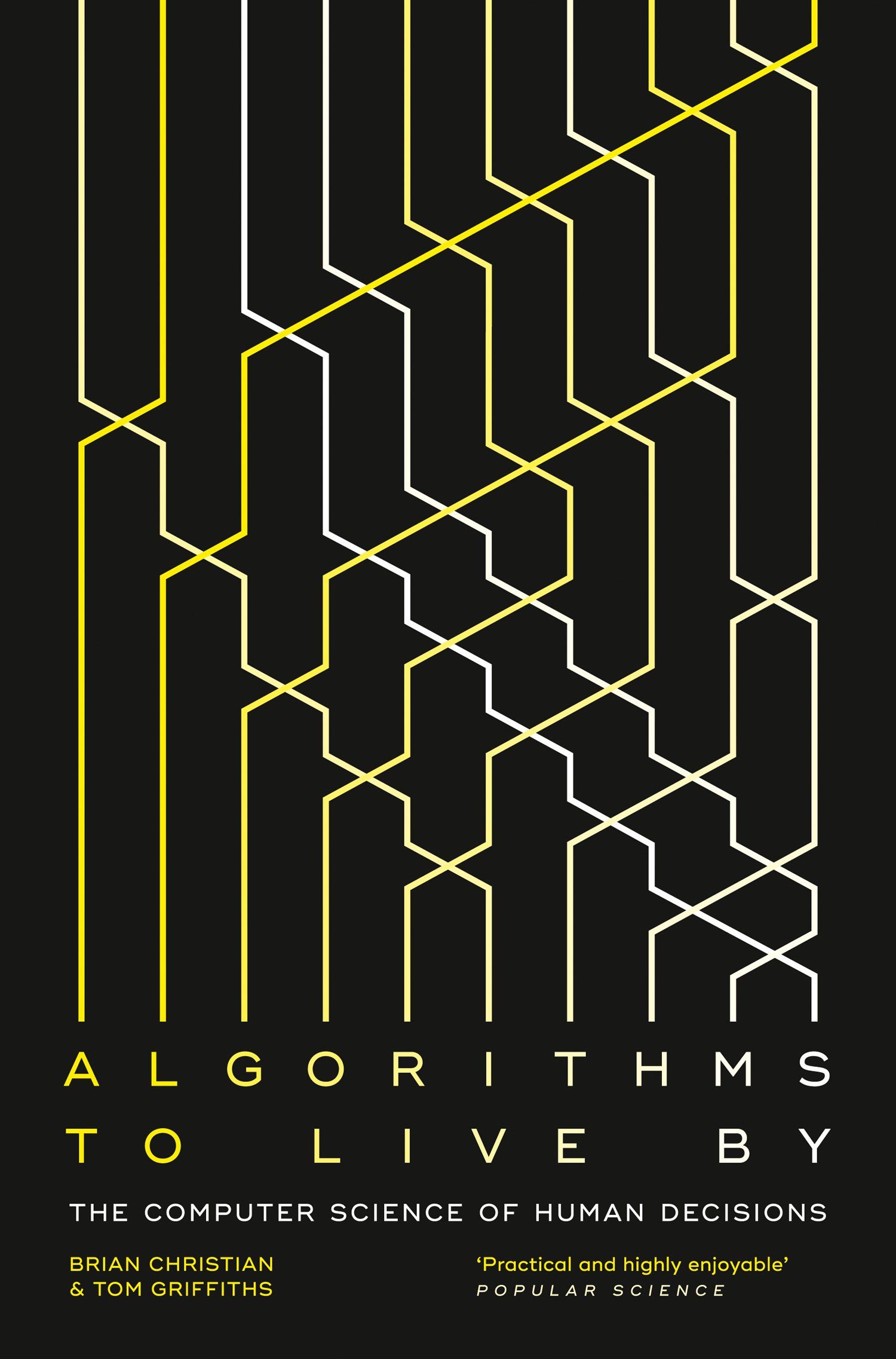